Professor of Surgery
Chair of Surgery
Professor in Integrative Immunobiology
Professor in Pediatrics
Core Faculty in Innovation & Entrepreneurship
Member of the Duke Cancer Institute
Email: surgery-lta@duke.edu
Overview
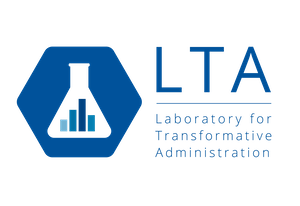
The Laboratory for Transformative Administration (LTA) uses data science methods on health system data to construct testable hypotheses around health system quality, cost, and operations and then implement solutions to improve resource allocation and quality of patient care. Put succinctly; we remove systemic barriers to surgical care.
The LTA utilizes health system data to address operational challenges that affect clinical care and have broader enterprise-wide impact. Our lab consists of attending physicians, administrators, clinical informaticists, engineers, data scientists, resident physicians, and other learners and researchers. We leverage our inter-professional team with broad technical skills and experiences and exist at the crossroads of strategic academic, clinical, and operational partnerships.
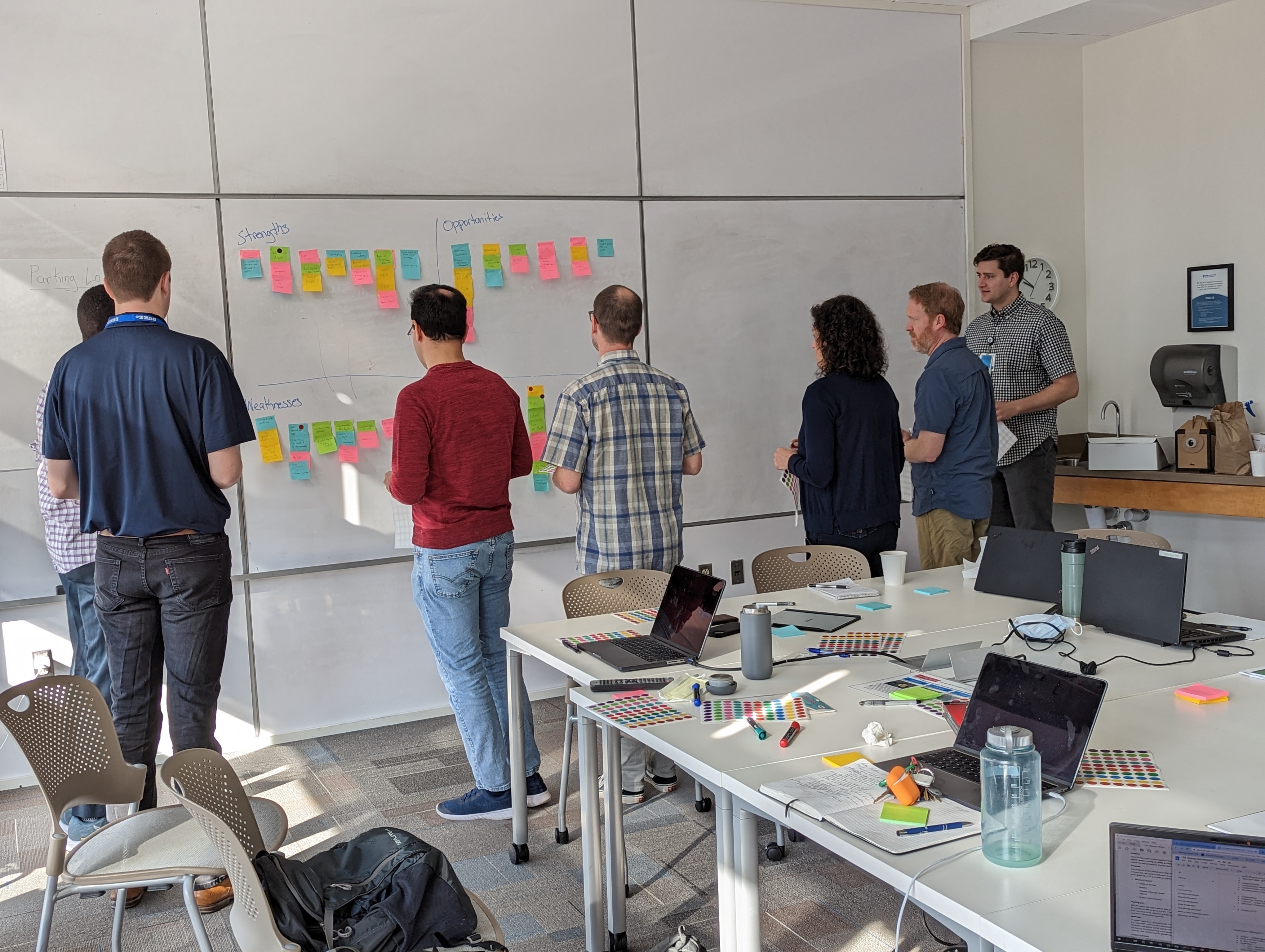
Lab Members
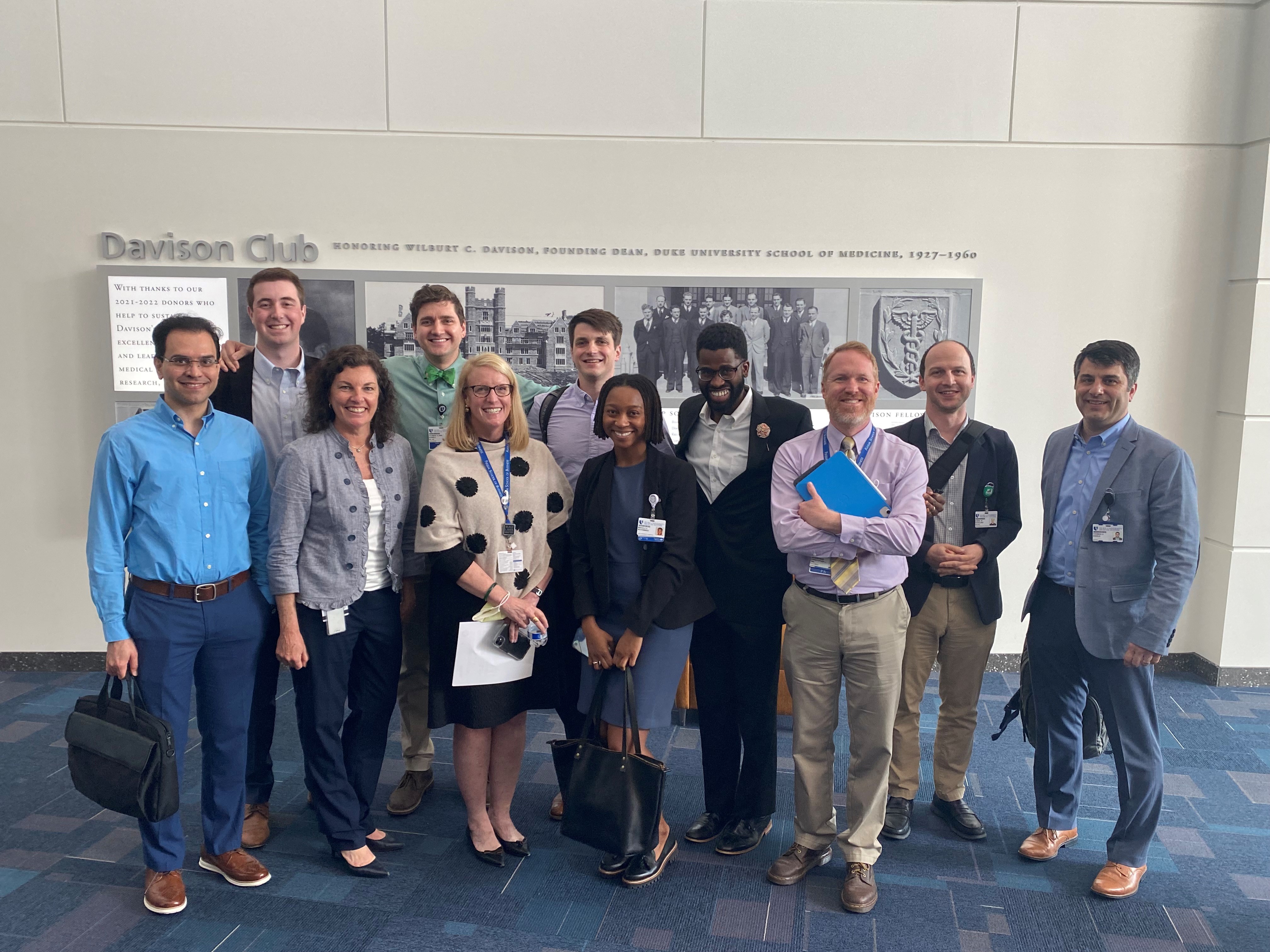
Principal Investigator
Co-Investigator, Medical Director
Co-Investigator, Lead Data Scientist
Operational Lead
Lab Members
Ursula Rogers
Clinical Informaticist
Hamed Zaribafzadeh
Data Scientist
John Rollman
Data Scientist
Chris Vail
Clinical Informaticist, MaestroCare Provider Champion, Trauma Physician Assistant
Makala Muhammed
Management Engineer
T. Clark Howell, MD
Resident Physician, Post-Doctoral Research Fellow
Fola Omofoye, MD
Researcher
Max Sumner
Medical Student, Business Student
Projects
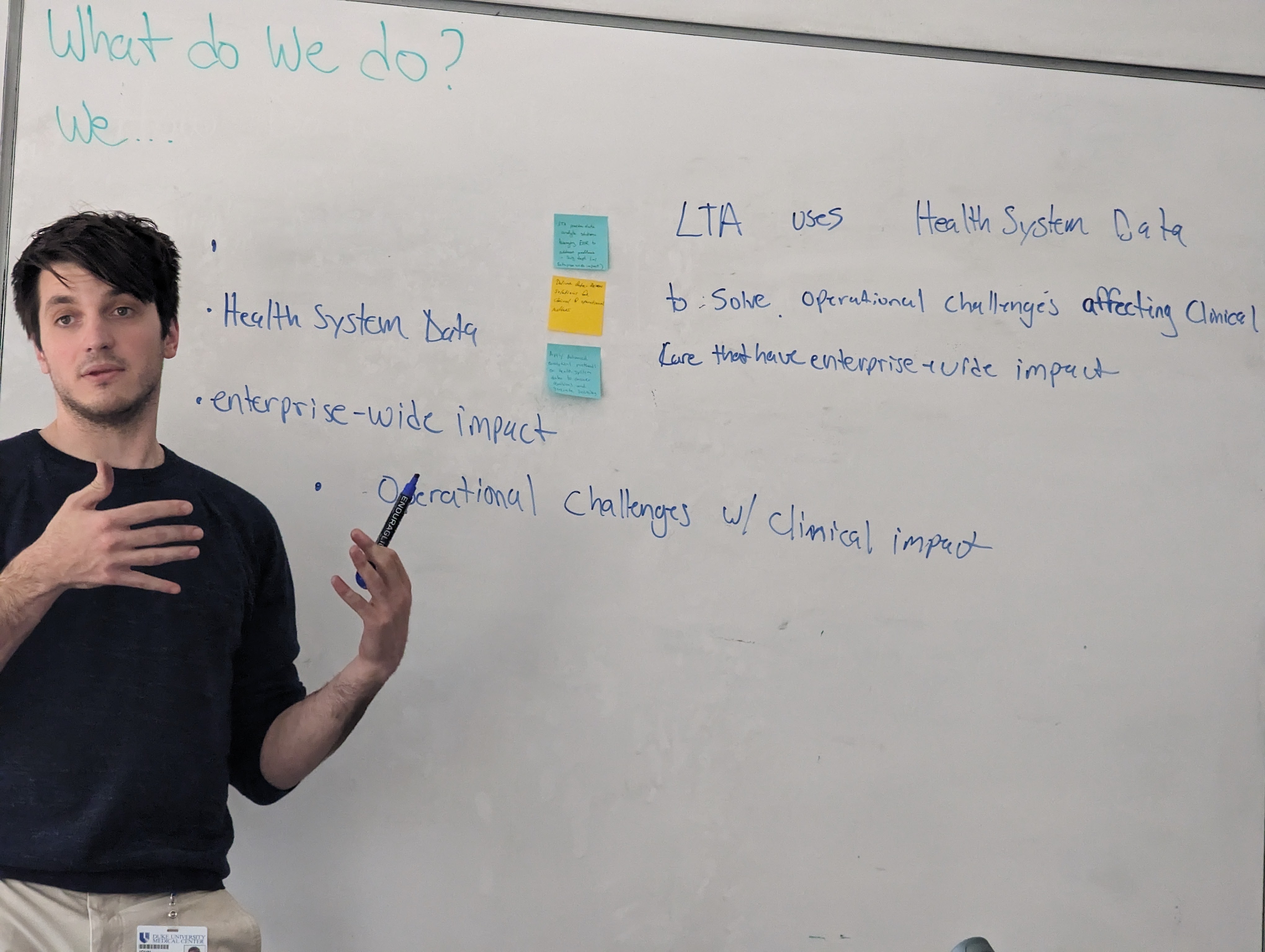
Developed an operational workflow and deployment framework that is being used every day to predict surgical case length at time of case posting for more efficient use of surgical resources.
- Zaribafzadeh, Hamed MS; Webster, Wendy L. MA, MBA; Vail, Christopher J. PA-C, MMCi; Daigle, Thomas BA; Kirk, Allan D. MD, PhD; Allen, Peter J. MD; Henao, Ricardo PhD; Buckland, Daniel M. MD, PhD. Development, Deployment, and Implementation of a Machine Learning Surgical Case Length Prediction Model and Prospective Evaluation. Annals of Surgery, June 2, 2023. | DOI: 10.1097/SLA.0000000000005936
- Garside, N., Zaribafzadeh, H., Henao, R. et al. CPT to RVU conversion improves model performance in the prediction of surgical case length. Sci Rep 11, 14169 (2021). https://doi.org/10.1038/s41598-021-93573-2
- Lam SSW, Zaribafzadeh H, Ang BY, Webster W, Buckland D, Mantyh C, Tan HK. Estimation of Surgery Durations Using Machine Learning Methods-A Cross-Country Multi-Site Collaborative Study. Healthcare. 2022; 10(7):1191. https://doi.org/10.3390/healthcare10071191
- Post-operative: Utilizing the deployed framework of the operative case length project we are developing a more accurate method to predict the post-operative inpatient length-of-stay at time of case request, which will assist discharge resource planning.
- Manuscript in review
- PACU: Utilizing the deployed framework of the operative case length project we are retooling the model to predict PACU LOS. Anticipating PACU LOS will allow schedulers to more efficiently sequence cases to match staffing needs as well as optimize bed flow.
- Surgical ICUs: Expanding on the inpatient length-of-stay at time of case posting work, a surgical ICU specific LOS model is being developed. The model predicts an initial ICU LOS at time of posting to aid bed flow and scheduling as well as an intra-ICU daily prediction utilizing rich physiological data collected during the ICU stay. The daily model will help physicians quantify a patient's trajectory and anticipate resource needs to improve patient care and flow.
Developed a model which predicted the need for surgical or conservative management at time of CT results in the acute care setting to improve surgical resource prioritization. Model uses clinical information available in the EMR at time of clinical decision.
In an effort to improve the quality of information available at case posting, common OR procedures were analyzed and their CPTs algorithmically grouped to create consistent pre-selected plain language posting options.
The posting option automatically populate the case log with the correct CPTs being performed reducing variation of information across same procedures and reducing post-hoc workload to correct information.
Operative, clinical quality, and direct hospital cost data were merged to create a scorecard report for physicians to evaluate their value-based-care performance and how they compare vs their peers.
Hernia and Cholecystectomy procedures were chosen as pilots for this effort.
Collecting, organizing, and employing data to determine patient eligibility for transplant, leading to improved equity in access to care, increased transparency in the transplant selection process and improved value for patients and clinicians.
Consortium for the Holistic Assessment of Risk in Transplant (CHART):
- Norine W. Chan, Mary Moya-Mendez, Jacqueline B. Henson, Hamed Zaribafzadeh, Mark P. Sendak, Nrupen A. Bhavsar, Suresh Balu, Allan D. Kirk, Lisa M. McElroy, Social determinants of health data in solid organ transplantation: National data sources and future directions, American Journal of Transplantation, Volume 22, Issue 10, 2022, Pages 2293-2301, ISSN 1600-6135, https://doi.org/10.1111/ajt.17096.
To improve quality and decrease cost, health systems are seeking to perform more ambulatory surgeries. However, there is no reliable and generalizable model that predicts which patients and which surgeries are appropriate for ambulatory venues.
Our aim is to create an ensemble model which predicts the optimal safe surgical venue.
Utilizing the more accurate surgical-case-length prediction results we are developing an optimization scheme to determine the most efficient OR schedules that allow the greatest number of procedures per day, as well as adaptive schedules that can compensate for day-of changes.
Developed models to evaluate patients' overall and recurrence-free survival after liver transplantation using pre- and post-transplant data
Exploring if adding automated clinical image analysis to already deployed LTA models improves sensitivity or specificity of their designed outcome and then how to implement and deploy the augmented models into clinical workflow.